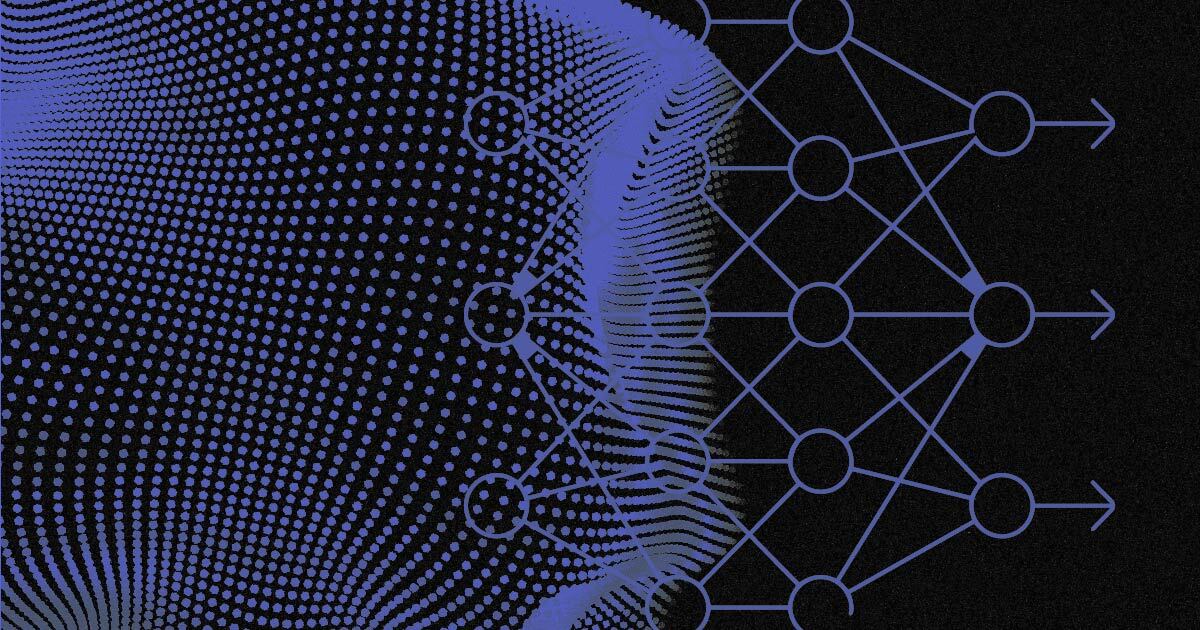
Deep learning (DL) has emerged in the past few years as a method for solving some of life sciences’ most complicated “big data” problems. These automated algorithms can extract insights from mountains of sequencing or imaging data much more rapidly and efficiently than humans can do manually. By deploying DL techniques to analyze complex volumes of data, such as those produced by the cell and gene therapy field, researchers can keep pace with the breakneck speed at which data is being generated. Genomics, for instance, is projected to equal or surpass social media, video, and other data-intensive areas in data generation and analysis within the next decade.1
Take a closer look at some of the obstacles in the big data “life cycle,” how deep learning can help, and 10 practical tips for implementing it in your cell and gene therapy research, development, and manufacturing in our infographic below.
The life sciences have never been more data-intensive. Biotechnology, pharmaceuticals, and medicine are now relying on mountains of sequencing, imaging, and modeling for research, clinical translation, and product development.
Cell and gene therapies are no different: Big data and powerful computational tools are solving complex discovery, development, and manufacturing problems.
Advancing Discovery
From mitigating off-target CRISPR-Cas editing to optimizing viral vector design, several unsolved problems are holding back cell and gene therapy discovery programs. Machine and deep learning algorithms are being employed to predict better gene editing outcomes during guide RNA design and reduce the immunogenicity of adeno-associated viral vectors.1
Driving Development
Developing and optimizing cell therapies relies on carefully interrogating the genetics and transcriptional activity in specific subpopulations. Single-cell sequencing has been used for cell therapy analysis, yet noisy and high-dimensional data is challenging to manage and requires extensive computational knowledge to troubleshoot. Deep learning models are increasingly applied to single-cell RNA-Seq data to bring deeper insights, more consistency, and improved efficacy and safety to emerging cell therapies.2
Accelerating Manufacturing
Cell and gene therapies undergo complex manufacturing processes that require skilled personnel, large-scale bioreactor systems, and complex culturing conditions, making them prone to error and batch-to-batch variability.
Automated equipment and deep learning algorithms, trained to monitor critical quality attributes, can help standardize processes and make manufacturing cell and gene therapies more consistent and reproducible.3
Genomics generates an astronomical amount of data, amounting to 1 zetta-bases/year of data, resulting in 2 to 40 exabytes/year of stored sequences data. This is far too much to analyze manually, even with robust bioinformatics pipelines.4 Here are ten actionable tips for implementing deep learning for data analysis.
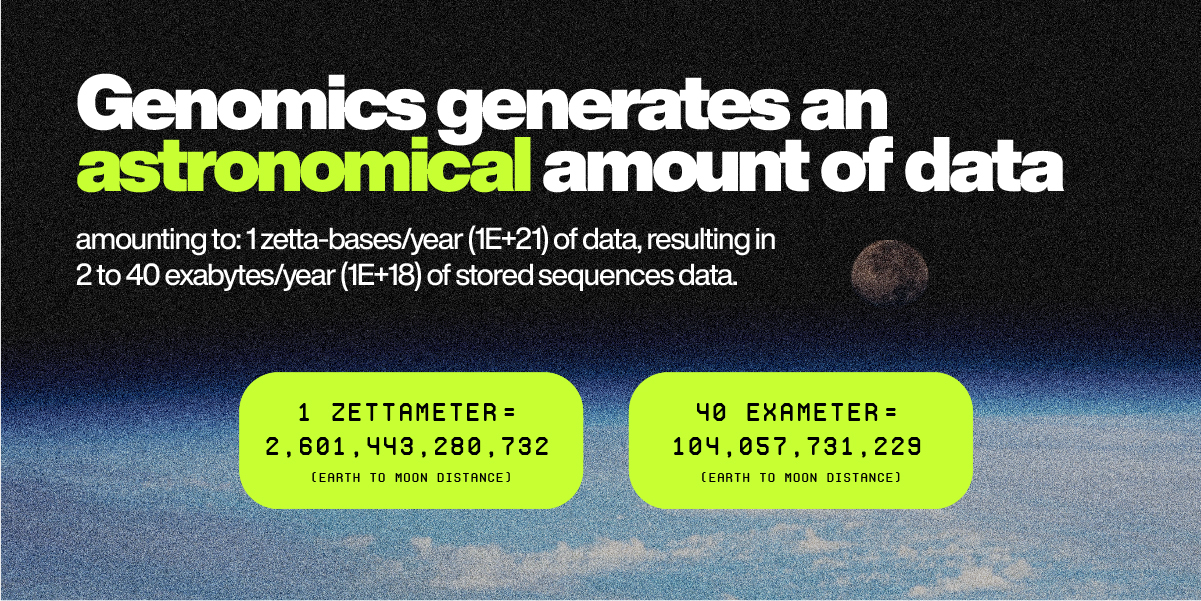
Tip 1: Use an Appropriate Method for Your Problem
With limited data or resources, non-deep learning models might be better suited to the problem you are trying to solve.
Tip 2: Establish Baselines Using Traditional Methods
Use well-tuned simple models to evaluate the performance of a deep learning model.
Tip 3: Make Sure You Understand the Complexities of Training
Ensure robustness and reproducibility in training by using established best practices.
Tip 4: Know Your Data and Your Questions
Understand the context and peculiarities of the data and problem to avoid pitfalls.
Tip 5: Select a Sensible Architecture
Let the problem inform network design and avoid reinventing the wheel.
Tip 6: Optimize Hyperparameters Extensively and Systematically
Systemic and extensive optimization of hyperparameters is vital for good results.
Tip 7: Mitigate Overfitting of Deep Neural Networks
Hold-out test data, regularize, and be aware of biological non-independence to prevent overfitting.
Tip 8: Maximize Transparency and Interpretability
Understanding how and why a model works is important for gaining biological insights.
Tip 9: Avoid Over-Interpretation of Predictions
Scientific inferences derived from a trained model should be independently verified.
Tip 10: Prioritize Research Ethics in Your Research
Consider implications, comply with legal and institutional regulations, and don’t inadvertently share private data.
These 10 tips were adapted from a previous article, originally published by Lee et al. in PLOS Computational Biology.5
Want to get updates on emerging computational life science trends straight in your inbox?
Sign up for our newsletterReferences
- Huang K, Xiao C, Glass LM, Critchlow CW, Gibson G, Sun J. Machine learning applications for therapeutic tasks with genomics data. Patterns (N Y) 2(10):100328 (2021).
- Yan R, Fan C, Yin Z, Wang T, Chen X. Potential applications of deep learning in single-cell RNA sequencing analysis for cell therapy and regenerative medicine. Stem Cells 39(5):511-521 (2021).
- Doulgkeroglou MN, Di Nubila A, Niessing B, et al. Automation, Monitoring, and Standardization of Cell Product Manufacturing. Front Bioeng Biotechnol 8:811 (2020).
- Stephens ZD, Lee SY, Faghri F, et al. Big Data: Astronomical or Genomical?. PLoS Biol 13(7):e1002195 (2015).
- Lee BD, Gitter A, Greene CS, et al. Ten quick tips for deep learning in biology. PLoS Comput Biol 18(3):e1009803 (2022).
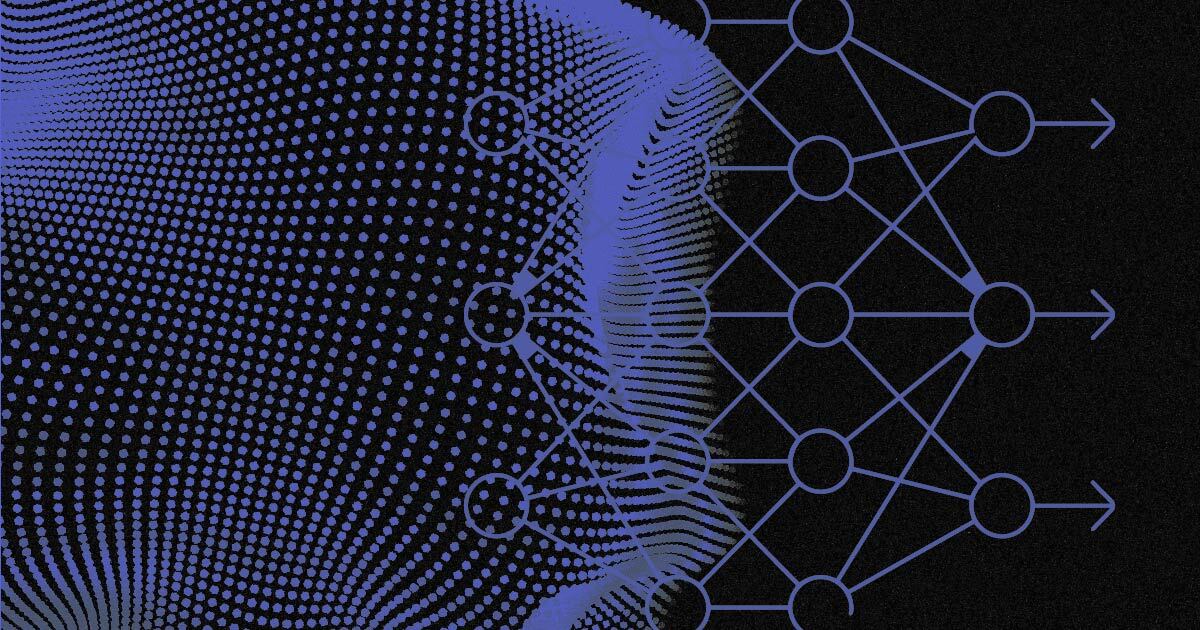