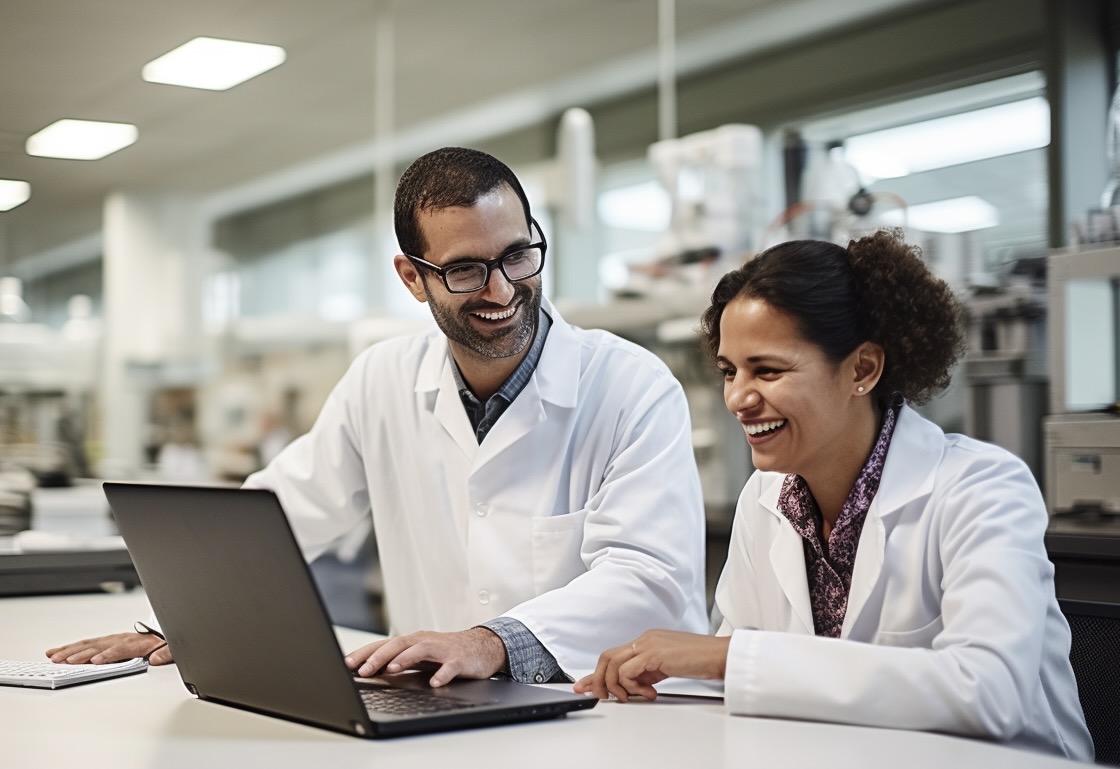
AAV-based therapies are powerful gene delivery tools, making it possible to treat genetic diseases, including rare ones, with a one-time cure. However, the design and engineering of vectors present significant challenges in the real world, leading to frequent failures in AAV drug development. There have also been several clinical holds during human trials of AAV gene therapies, some due to unexpected adverse events.1
Failed AAV drugs underscore the urgent need to improve vector designs to enhance efficacy and success rates and provide these potentially life-saving therapies to patients. Integrating AI-assisted experiments into regular laboratory assays and drug development pipelines offers a promising approach to overcoming these challenges and improving data reliability.
The Importance of AI in Leveraging the Potential of AAV Biological Data
There is so much biological data now that humans can’t manage and analyze it all.
If you think about any data-heavy industry – finance, manufacturing, security, healthcare, biotech, etc. – you can imagine a use case for AI. AI can also unlock the vast potential of analog data sources (paper notebooks, historical writings) rich in metadata and labels.2 Digitizing and analyzing this data can significantly improve experimental results.
In the life sciences, successful AI integration requires collaboration between researchers, data engineers, and AI scientists. This fosters a data-centric approach that merges data, statistical analysis, and hypothesis-driven experimental design. AI has enabled more efficient and insightful imaging and genomic investigation and has been implemented across diverse fields, such as proteomics, epigenomics, metabolomics, and drug discovery.3,4 Moreover, AI and data science augment biological lab research, enabling iterative refinement of research questions, data collection, model training, and publication.5,6 This ensures the best methods are used for answering specific questions.
AI tools like transformer-based language models analyze scientific literature and design chemical synthesis protocols, accelerating research methods, and are now available for AAV vector design. In addition, guidelines for reporting on the use of AI/ML are improving the reproducibility of its use in the life sciences.4
Establishing Trust in AI-Assisted AAV Research Approaches
While the early adopters of AI are experiencing its numerous benefits, there is a known large pool of skeptics in the biotech and biopharma fields.7 As an industry, we’re still learning how to use and trust these new AI technologies that have the potential to help scientists work more efficiently and free up time for more strategic thinking.8
Single-cell sequencing technology provides a relevant example: the advent of large-scale single-cell atlases and deep learning has transformed our understanding of cell states. However, existing computational methods that facilitate this analysis often lack biological interpretability. Recently, Lotfollahi et al. described ExpiMap, a new deep-learning architecture that addresses this by mapping cells into understandable components based on known gene programs, enhancing data interpretability and accuracy.9 By outperforming existing methods, ExpiMap underscores the crucial role of accurate computational tools in translating complex lab data into meaningful biological insights.
Validation of AI/ML in the wet lab is also essential in building trust in the life sciences. In the AAV research field, several reports have experimentally validated the findings of AI algorithms to improve the design and function of AAV vectors. For example, Bryant et al. used deep learning to predict the viability of 110,689 out of 201,426 engineered AAV2 variants, surpassing the diversity of natural serotypes.10 These predictions were confirmed experimentally. Another study by Eid et al. used an ML approach, Fit4Function, to design multi-trait AAV capsids.11 Models trained on screening data accurately predicted variant performance, with 89% meeting all criteria in lab validation, and demonstrated cross-species prediction accuracy.
Recently, Form Bio has validated its AI technology, FORMsightAI, by collaborating with leaders in the industry for experimentally validating that it can accurately and reproducibly predict AAV vector design problems and generate optimized designs that increase desirable characteristics and reduce undesirable ones.
These examples underscore ML's role in expanding the functional sequence space and advancing gene therapy through precise, experimentally validated predictions.
Leveraging AI for Future Success in AAV Research and Vector Design
AI-assisted experiments have enhanced the design and success of AAV gene therapies by mining vast amounts of data for critical insights. While there is still skepticism in the field, experimental validations in laboratories demonstrate AI's ability to predict and optimize AAV vectors, advancing gene therapy through precise, reproducible outcomes.
At Form Bio, we’ve developed In Silico Assays that use the primary DNA sequence of an AAV construct to produce:
- Highly comparable sedimentation curves to lab analytical ultracentrifugation (AUC) results with similar proportions for full and partial genomes
- Long-read sequencing data that are highly correlated for full vector genomes (r2=0.74) and partial vector genomes (r2=0.96)
These predictive computational assays enable the rapid screening and de-risking of AAV vector design, saving time and resources, and accelerating discovery.
AI Disclosure: Feature image was made with an AI image generator MidJourney.
Want to hear more about Form Bio’s AAV Partner Program?
Schedule Your Discovery Call TodayReferences
- Wills CA, Drago D, Pietrusko RG. Clinical holds for cell and gene therapy trials: Risks, impact, and lessons learned. Mol Ther Methods Clin Dev. 2023;31:101125.
- Pandey M, Arora M, Arora S, Goyal C, Gera VK, Yadav H. AI-based Integrated Approach for the Development of Intelligent Document Management System (IDMS). Procedia Comput Sci. 2023;230:725-736.
- Libbrecht MW, Noble WS. Machine learning applications in genetics and genomics. Nat Rev Genet. 2015;16(6):321-332.
- Walsh I, Fishman D, Garcia-Gasulla D, et al. DOME: recommendations for supervised machine learning validation in biology. Nat Methods. 2021;18(10):1122-1127.
- Fiorillo L, Mehta V. Accelerating Editorial Processes in Scientific Journals: Leveraging AI for Rapid Manuscript Review. Oral Oncol Rep. Published online May 21, 2024:100511.
- Boiko DA, MacKnight R, Gomes G. Emergent autonomous scientific research capabilities of large language models. Published online April 11, 2023. Accessed July 10, 2023.
- Ph.D DEF and PL. Is the biopharma industry right to be skeptical about AI? MedCity News. Published December 14, 2020. Accessed May 23, 2024.
- U.S. Department of Health and Human Services. Trustworthy AI (TAI) Playbook: Executive Summary. Published September 2021. Accessed May 23, 2024.
- Lotfollahi M, Rybakov S, Hrovatin K, et al. Biologically informed deep learning to query gene programs in single-cell atlases. Nat Cell Biol. 2023;25(2):337-350.
- Bryant DH, Bashir A, Sinai S, et al. Deep diversification of an AAV capsid protein by machine learning. Nat Biotechnol. 2021;39(6):691-696.
- Eid FE, Chen AT, Chan KY, et al. Systematic multi-trait AAV capsid engineering for efficient gene delivery. Published online December 22, 2022:2022.12.22.521680.
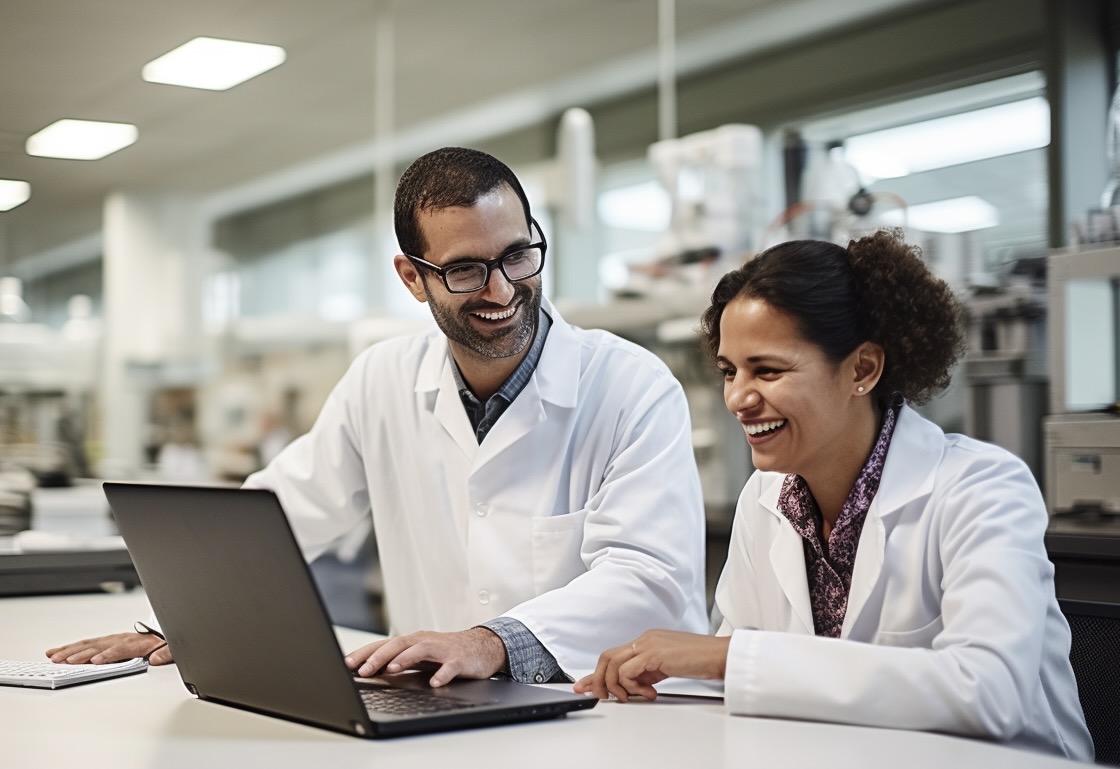