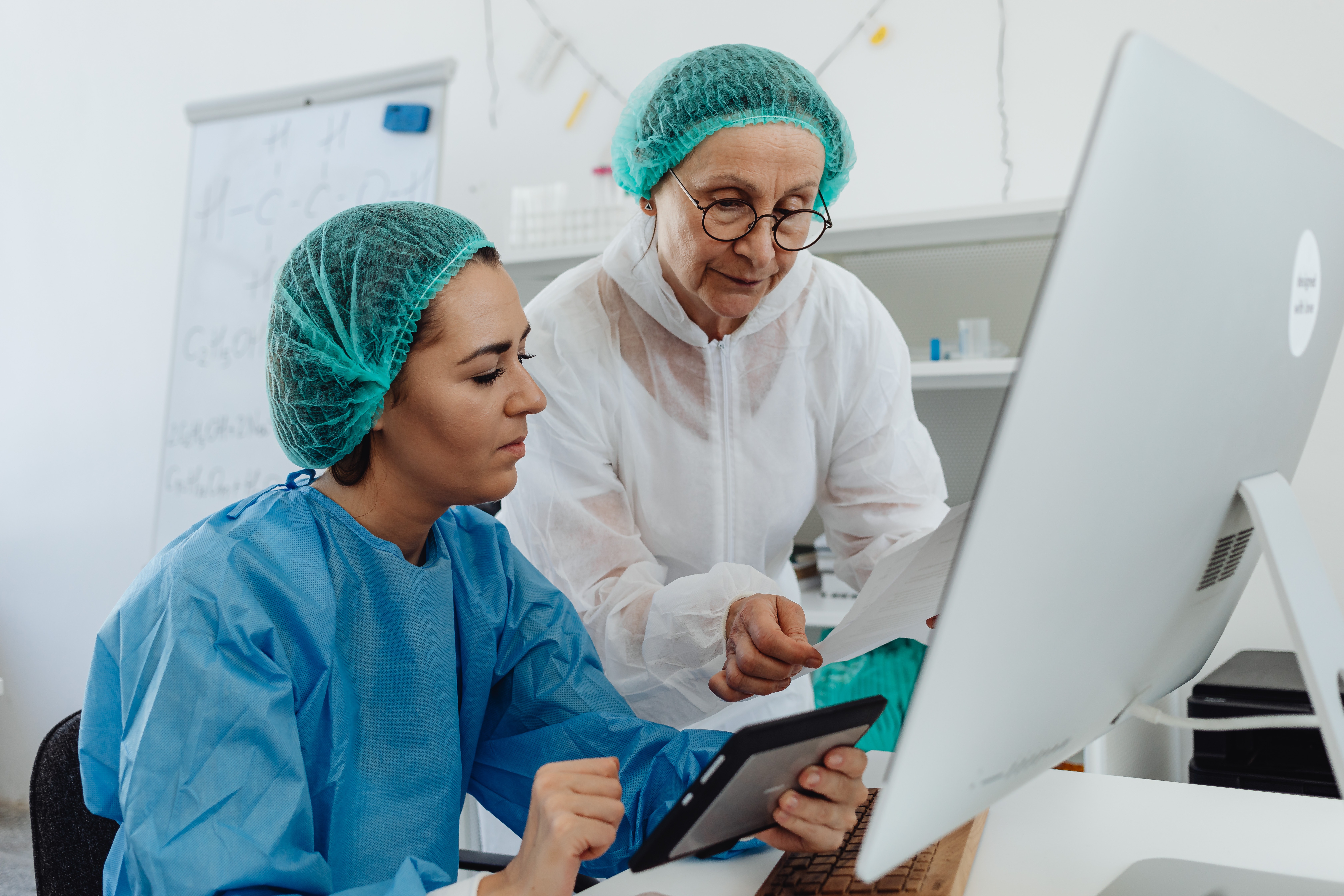
The ability to interpret data is crucial to advancing cell and gene therapy research. But raw data is rarely useful when it comes to gleaning insights. To effectively use data and communicate learnings, cell and gene therapy researchers need to present data in an accessible way.
This is why computational life science data visualization tools are critically important to cell and gene therapy research.
Why is Data Visualization so Important in Cell and Gene Therapy?
Visuals used in research were long limited to simple Excel pie or bar charts, displays generated by instrumentation, e.g. flow cytometry dot plots, or 2D images. But with larger data sets created by cutting-edge experimental techniques, data analysis visualization plays an increasingly central role in translating data into insights as well as communicating these insights. Data visualization is emerging as a subdiscipline as some scientists as more realize it is an essential tool for revealing insights buried in complex data.1
Data analysis and visualization using modern software tools is much more than just a way to make data look pretty, experiments have shown that humans recognize and process pictures more effortlessly than words and also find it easier to recall them - a phenomenon called “picture superiority effect”. 2
Studies have also shown that visuals help people gain insights through a four-step process by:
- Providing an overview – visualizations help grasping the big picture and honing in on the important data
- Adjusting – being able to interactively adjust the data visualization, e.g. through filtering, grouping, or sorting, helps to make sense of the data
- Detecting patterns – visualizations help with seeing trends, detecting outliers or finding structure in a dataset that aren’t obvious from looking at raw data
- Matching mental models – visual representation makes it easier to understand the data by linking it to real-world knowledge
Data analysis visualization serves another important purpose: it increases the user’s interaction with the data, which is the best way to generate insights.
Current Uses of Data Visualization in Cell and Gene Therapy
Data visualization is used broadly in the life sciences and cell and gene therapy. Here are some examples of applications that are particularly important.
Genome and Sequence Annotation
Raw DNA sequences are nothing but long strings of the letters ACG and T. To make sense of the data, annotations that identify e.g. exons, introns, genes, or regulatory regions are needed. Visualizing sequences as linear representations with the annotations is the most intuitive way to present that data.
Sequence Analysis
Without visualization tools it is impossible to compare sequences, e.g. from different individuals. Aligning sequences with conserved segments highlighted is a good way to visualize alignment, similarities and differences.
RNA-Seq Analysis and Expression Profiling
Interpreting the high-dimensional data sets from RNA-seq experiments and reliably detecting differentially expressed genes remains a formidable challenge. Heat maps have been used since the early days of RNA analysis using microarrays but as data sets get ever larger novel visualization tools, such as gene expression plots, network maps, volcano plots and others are needed 3.
Visualization tools can also highlight patterns and problems they may not detect with standard models, such as normalization issues, differential expression designation problems, and analysis errors6.
Protein Structure
Generating 3D renderings of proteins allows researchers to gain insight into the molecular mechanisms underlying cellular biochemical processes. The Protein Data Bank archive7, which makes most of these structures available is a valuable resource that has enabled fast advances in visualization of molecular graphics8.
Systems Biology
Systems biology uses mathematical analysis and computational models to describe
biological systems. Visualizations are key to making sense of and communicating these complex data. In addition to well-established pathway maps network graphs are important tools to visualizing systems biology data sets.
In these and many other research areas computational life science data visualization of large amount of complex information facilitates data mining and analysis.
Types of Visualization
Visualizations address one of the key challenges of data-heavy modern life sciences: they allow researchers to benefit from the torrent of data without being overwhelmed by it. Here is an overview of the most common types of data analysis visualizations:
Heatmaps
For visual encoding of data matrices using color. They make it easier to detect patterns in high-density data sets. Heatmaps are used extensively in expression to visualize relative changes in gene expression.
Network graphs
A classical visualization tool used to show complex relationships of molecular interactions, e.g. protein interactions, metabolic signaling, and gene regulatory relationships.
Scatter plots
Present the relationship between two variables in a data-set by representing data points on a two-dimensional plane. They are used for large sets of numerical data where each set each comprises a pair of values
Volcano plots
A special type of scatter-plot that is used to identify changes in large data sets composed of replicate data. They are commonly used to display the results of RNA-seq or other omics experiments. They enable the quick visual identification of genes with large fold changes that are also statistically significant.
Dendrograms
Tree diagram used to illustrate the arrangement of the clusters produced by hierarchical clustering. In computational biology dendrograms are used to illustrate the clustering of genes, proteins, metabolites or samples.
Box Plots
A visualization of statistical data based on the minimum, first quartile, median, third quartile, and maximum score. They are used e.g. in gene expression analysis to visualize the distributions of gene expression values across samples 9.
Histograms
Graphical representations of data points organized into user-specified ranges. They condense a data series into an easily interpreted visual by taking many data points and grouping them into logical ranges or bins. In research they are used
Current Challenges with Data Visualization
Computational life science data visualization has come a long way over the last decade, but challenges remain that future innovations will have to address. Challenges include:
Better tools for Interpreting the high-dimensional data sets
High-dimensional data sets, e.g. from RNA-seq experiments are typically displayed in the form of heatmaps. However, the bigger the data sets the worse optical illusions become making it impractical to display all data in one large heatmap 8. New, preferably interactive visualization tools might be able to address these short-comings.
3D Visualization
Being able to view a molecule in 3D is particularly critical when studying proteins and their interactions. Technologies such augmented reality or virtual reality which were developed for other applications, e.g. gaming, can help develop highly accurate 3D visualizations of proteins.
Generating ease of use interactive visualization tools
Interactive visualizations allow users to deeply engage with the data and foster learning. For scientists the ability to generate interactive graphs that can be shared with colleagues who can then “play” with the data even if they don’t know how to code would be a valuable tool. This way computational and bench scientists could collaborate more easily and shorten cycle times.
Data Visualization Resources for Cell and Gene Therapy
Here is a short list of data analysis visualization tools:
Jmol - A free and open source viewer of molecular structures with features for chemicals, crystals, materials and biomolecules10.
Cytoscape - Cytoscape is an open source software platform for visualizing complex networks and integrating these with any type of attribute data. Cytoscape is used in other fields, but also supports many use cases in molecular and systems biology, genomics, and proteomics 11.
RasMol - An open source, free program assists in visualizing and analyzing the biological macromolecules of interest 12.
CBioPortal for Cancer Genomics - provides visualization, analysis and download of large-scale cancer genomics data sets 13.
UGENE free open-source cross-platform bioinformatics software for dot plot & chromatogram visualizations 14.
Conclusion
As data sets get bigger and more complex, visualization becomes increasingly more important for cell and gene therapy scientists as they use this data to answer important questions and collaborate with colleagues.
While many advanced tools exist to visualize everything from the 3D structures of proteins to gene expression data, more, ideally interactive tools are needed. In addition, these tools need to be easy enough to use so not just experts can generate and interact with them but every researcher is enabled.
Better visualizations accelerate progress and foster dissemination and knowledge transfer among colleagues as well as a broader audience.
Want to learn how Form Bio empowers every scientist to create powerful data visualizations?
Schedule Your Demo TodayReferences
- O’Donoghue, S.I. Grand Challenges in Bioinformatics Data Visualization. Front. Bioinform. (2021).
- Defeyter M A. The picture superiority effect in recognition memory: A developmental study using the response signal procedure. Cogn. Dev. (2009).
- Bonner E. Object representation in the human brain reflect the co-occurance statistics of vision and language. Nat Commun 12, 4081 (2021).
- Epstein, R.A. Scene perception in the human brains. Annu Rev Vis Sci. 5:373-397 (2019).
- Wills, Q.F. Single-cell gene expression analysis reveals genetic association masked in whole-tissue experiments. Nat Biotechnol. 31:748-752 (2013).
- Rutter L. Visualization methods for differential expression analysis. BMC Bioinformatics (2019)
- Worldwide Protein Data Bank , wwPDBFoundation. Accessed September 12, 2022
- O’Donoghue, S.I. Visualization of Biomedical Data. Annu. Rev. Biomed. Data Sci. (2018)
- Zhou G. NetworkAnalyst 3.0: a visual analytics platform for comprehensive gene expression profiling and meta-analysis. Nucleic Acids Res. (2019)
- Jmol: an open-source Java viewer for chemical structures in 3D. SourceForge. Accessed September 12, 2022
- Cytoscape, . Cytoscape Consortium. Accessed September 12, 2022
- RasMol. SourceForge. Accessed September 12, 2022
- cBioPortal. Accessed September 12, 2022
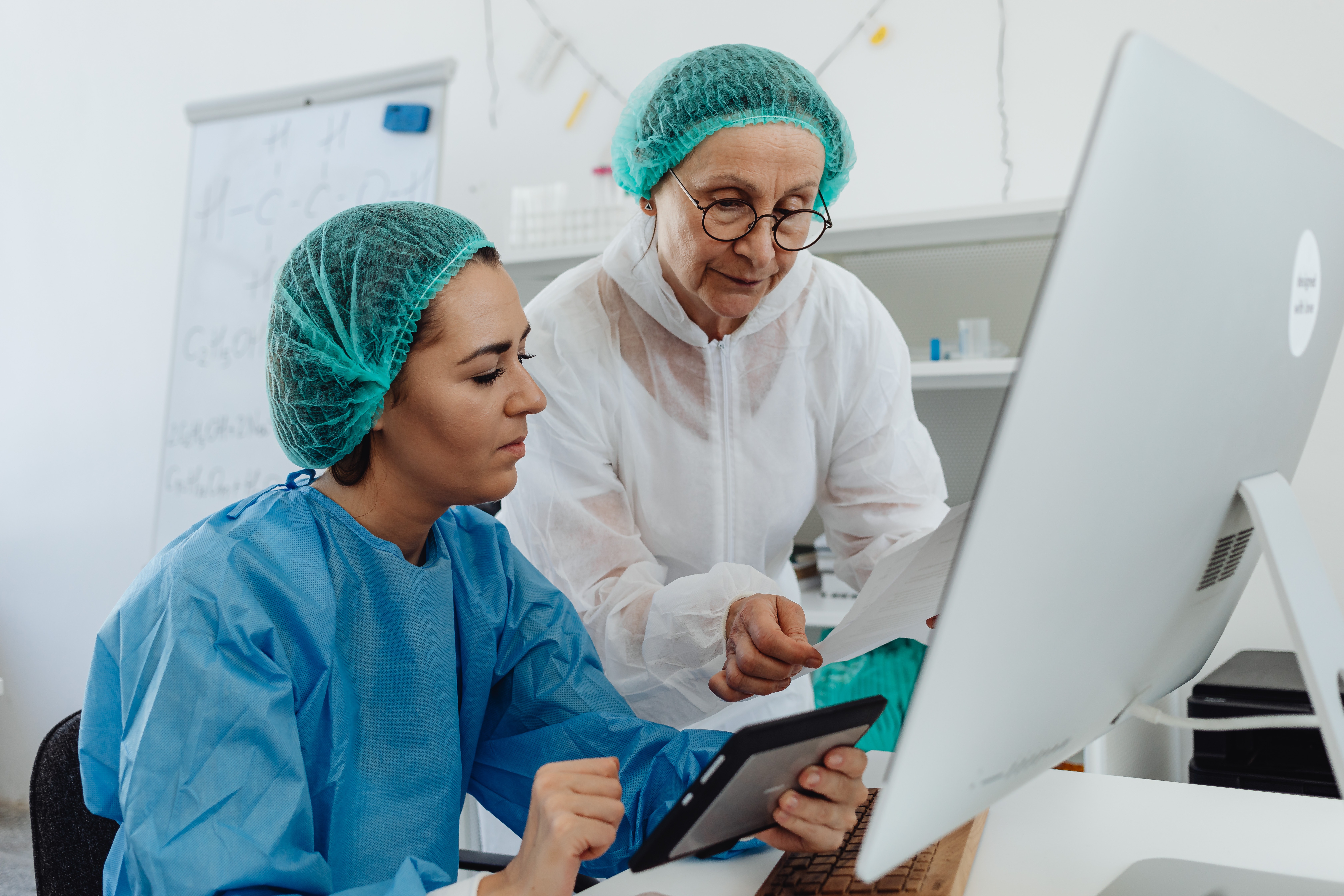