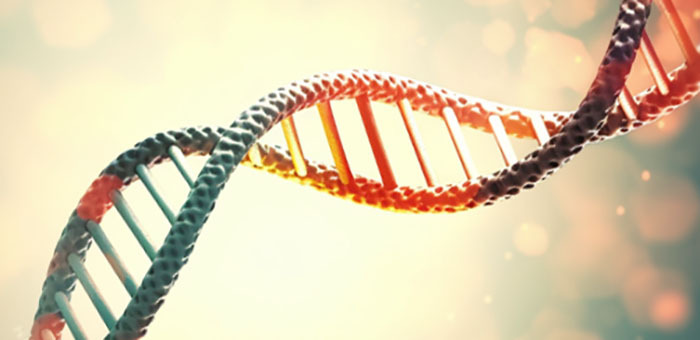
By harnessing the power of genetic engineering, gene therapies aim to correct or replace faulty genes within an individual's cells, offering the potential of one-dose cures for a broad range of diseases. Thus far, the FDA has approved gene therapies for retinal dystrophy, spinal muscular atrophy, Hemophilia B, bladder cancer, and, most recently, Duchenne muscular dystrophy and Hemophilia A, marking a major advancement this year since the first gene therapy FDA approval in 20171-7. Moreover, a whole new class of gene therapy has opened up in the UK for the first CRISPR drug ever to be approved by a regulatory body.
Alongside these landmark advancements in medicine come a heap of discovery, development, manufacturing, and clinical challenges for biopharmaceutical teams to overcome. Safety concerns in gene therapy clinical trials were responsible for the large spike in clinical holds in 20218. Biomanufacturing these complex biologics remains challenging to scale while maintaining high-quality production processes. With the amount of activity in the genetic engineering and gene therapy space, many companies are (or will be) attempting to solve these problems to bring safer and more effective therapies to market faster.
With all of the recent breakthroughs in AI in gene therapy, large language models and transformer architecture hold significant promise for solving the many challenges that gene therapy developers face9,10. In the following blog, we’ll discuss the benefits of AI, applications of AI in gene therapies and genetic engineering, and the current and long-term practical challenges for implementing AI into your gene therapy development workflow.
The Rise of AI Use For Genetic Engineering and Gene Therapy
Over the past decade, the pipeline of gene therapies that use powerful genetic engineering tools has expanded significantly. In December of 2023, the first gene therapy that uses the genetic engineering tool, Cas9, was approved.11 In 2024, it’s predicted that there will be 12 approval decisions made regarding gene therapies, several of which target rare diseases12,13.
Despite these current and future clinical successes, significant improvements are required. First, the cost of FDA-approved gene therapies poses a challenge to accessibility for patients14. Jennifer Doudna, who in part discovered CRISPR/Cas systems and leads the therapeutic applications of the gene editing tool, calls the global delivery of CRISPR-based therapies “unrealistic"15. Second, the adeno-associated virus (AAV) vector-based gene therapies, the favored vector for gene delivery, are largely considered safe, yet there are still challenges with managing adverse events and preventing death. In 2021, four boys died in a Phase I/II trial of Astellas Pharma’s resamirigene bilparvovec, an AAV-based gene therapy for X-linked Myotubular Myopathy (XLMTM)16,17.
As an industry built to help people live longer and healthier, we need better genetic engineering and gene therapy outcomes.
The Applications of AI in Gene Therapy Development
AI can potentially drive transformative advancements in several pre-clinical and clinical gene therapy development stages.
Personalized Medicine
By analyzing genomic data and identifying disease-associated genetic variations, AI can contribute to personalized treatment strategies. It assists in designing tailored gene therapy approaches and predicting therapeutic outcomes for individuals. Furthermore, AI enables real-time monitoring of patient data, facilitating adaptive therapies. It analyzes gene expression profiles, clinical parameters, and treatment responses, providing insights for personalized adjustments to treatment plans.
Target Identification and Validation
AI algorithms can identify potential gene targets and validate their therapeutic relevance by analyzing large-scale genomic and molecular datasets. This enables the discovery of novel gene candidates and the evaluation of their suitability for gene therapy interventions.
Viral Vector Design Optimization
AI algorithms can be used to enhance the viral vector capsids for improved transfection efficiency, tissue targeting, and other factors.
Optimizing Viral Vector for Manufacturing
Computational modeling and simulation are used to gauge the efficiency of encapsulating complete constructs within capsids to ensure the greatest predicted likelihood of manufacturing success while providing cost efficiency.
Clinical Predictions
AI excels in gene therapy efficacy and safety prediction by integrating diverse datasets. It constructs predictive models to assess treatment outcomes and optimize protocols, leading to improved patient responses.
Biomarker Predictions
AI uncovers meaningful patterns and potential biomarkers through genomics and molecular datasets analysis. This discovery process aids in identifying novel therapeutic targets, developing patient stratification approaches, and establishing biomarkers to monitor treatment effectiveness.
What Are The Current Challenges of Using AI in Gene Therapy Development?
While there is considerable promise for AI in gene therapy research, it is important to acknowledge the significant challenges that still need to be overcome. Many problems in AI stem from the quality of the data used. By addressing these challenges, we can ensure that the current partnership between AI and gene therapy development drives sustainable change. With continued advancements, research, and collaboration, we can unlock the full potential of AI in revolutionizing gene therapy and improving patient outcomes.
Limited and Heterogeneous Data
AI algorithms typically require large amounts of high-quality data to train and produce reliable results. Gene therapy data is often limited due to the rarity of certain diseases and the high costs associated with data collection. Additionally, the data obtained from different sources may be heterogeneous regarding formats, quality, and labeling standards. These variations can make training accurate and reliable deep learning models challenging. The solution to this “data scarcity” problem is the strategic curation of gene therapy-specific datasets that can be used for training the next generation of niche AI algorithms.
Extensive Compute Power
The requirement for extensive computational power and large volumes of data to train AI systems stands as one of the most significant challenges. Regrettably, these resources are frequently accessible only to industry giants, leading to an imbalance within the AI development ecosystem. This disparity jeopardizes the fundamental scientific research necessary to achieve our ambitious technological goals. Resolving this imbalance calls for a substantial investment in AI from the public sector.
Data Privacy and Access
Gene therapy data, especially patient-specific genomic data, is highly sensitive and subject to privacy regulations. Balancing the need for data access while respecting privacy constraints is a significant challenge. Access to diverse and representative datasets is crucial for training robust models, but strict regulations and consent requirements can limit data availability.
Labeling and Annotation Challenges
Deep learning models require labeled data for supervised training. However, labeling gene therapy data can be complex and time-consuming. Expert knowledge is often required to annotate and interpret genomic sequences, which may limit the scalability and efficiency of data labeling processes.
Interpretable Models
Gene therapies involve complex biological processes, and understanding the underlying mechanisms is crucial for successful treatment. Deep learning models, particularly deep neural networks, are often considered black boxes, making it difficult to interpret their predictions and understand the reasoning behind them. Developing interpretable models that provide insights into the biological processes will enhance the trust and acceptance of AI within the life science industry.
Generalization Across Datasets
Deep learning models trained on one dataset may struggle to generalize well to other datasets due to differences in data distribution, batch effects, or technical variations. Achieving robust and generalizable models for gene therapies requires careful consideration of dataset biases and developing strategies to minimize these biases during model training.
Data Integration and Fusion
Gene therapy research often involves multiple data types, including genomic, clinical, imaging, and more. Integrating and fusing heterogeneous data sources to create a comprehensive dataset can be complex. Developing effective techniques to combine and leverage different data modalities is essential for building accurate and holistic deep learning models.
Addressing these challenges requires collaboration between genomics, bioinformatics, and AI experts, along with advancements in data sharing frameworks, privacy-preserving techniques, and interpretability methods. Overcoming these obstacles will enable the development of robust deep learning models that can accelerate advances in gene therapies.
The Future of AI in Gene Therapy: Solutions on the Horizon
With several long-term solutions listed below, as an industry, AI-enabled biotechnology has the potential to bring about the approval of safer, more effective, and accessible gene therapies for a wide range of diseases.
Platform Approaches
Streamlining gene therapy manufacturing is a critical step in managing development costs and accessibility for patients18. Transitioning to a platform approach in gene therapies means shifting from a one-off or bespoke approach to a more standardized and scalable model for developing and manufacturing these therapies. This approach involves developing a set of common processes, technologies, and infrastructure that can be applied to multiple gene therapy products and streamlines communications with the FDA to demonstrate gene product content and satisfy efficacy and safety requirements needed for approval.
Analytical Standardization
Efficiency and scalability come from standardization. Collectively, the gene therapy industry needs to communicate the precise composition and attributes of these therapies to regulatory bodies using validated methods for product characterization.19 Though standardization is challenging, consistency in measuring critical quality attributes (CQAs) will facilitate regulatory compliance and increased safety.
Transparency in Validating In Silico and In Vitro Work
In the life sciences, validation and reproducibility only come with transparency. AI applications in the life sciences are no different: Transparency in the development and training of an algorithm is the only way to ensure effective utilization and reduce skepticism. Without this, the improvement of models will slow, and so will the integration of AI into project workflows. Establishing a transparent validation process that incorporates both in silico and in vitro methods is important. These combined efforts strengthen the groundwork on which the future of AI-driven progress in healthcare and life sciences can securely rely.
Data availability and data generation standards
The absence of standardized data poses a significant hurdle as it hampers the ability to validate and reproduce results. Establishing robust data generation standards is essential to overcome this reproducibility problem.
Ethics and regulatory considerations
Addressing ethical and regulatory concerns becomes paramount as AI becomes more integral to gene therapy research, development, and manufacturing. Safeguarding patient privacy, ensuring informed consent, and establishing guidelines for responsible AI usage are essential for maintaining trust and ethical standards.
Converting data into valid insights
Extracting meaningful insights from the collected data is a critical challenge. Developing advanced algorithms and methodologies that can effectively process and interpret complex genomic and other ‘omics data is necessary to derive valid and actionable insights.
Internal infrastructure development
Establishing robust and secure internal systems, such as federated data systems, can facilitate collaborative research and data sharing while ensuring data privacy and security20.
How Form Bio is Accelerating Gene Therapy with AI
Form Bio specializes in AAV gene therapy viral vector design optimization. Harnessing the power of AI in gene therapy, AAV development and manufacturing requires the development of a robust AI infrastructure specific to AAV applications and overcoming several hurdles21. At Form Bio, we’ve developed a AAV gene therapy product characterization and computational platform solution, FORMsightAI, to build custom in silico program to accelerate development and manufacturing.
FORMsightAI uses advanced and customizable AI models trained on AAV datasets so you can characterize drug products, compare and optimize different AAV construct designs, simulate bioreactor production, enhance the AAV capsid for improved transfection efficiency, tissue targeting, and other factors, and gauge the efficiency of encapsulating complete constructs within these capsids. Leveraging FORMsightAI early on in preclinical gene therapy development programs will ultimately speed up time to clinical trials, lower the costs of gene therapies, and get safe, efficacious therapies to patients faster.
FAQs
How is AI Used in Gene Therapy?
AI is used in various aspects of gene therapy to enhance the development, optimization, and implementation of treatments. Applications include target identification and validation, drug discovery and development, biomarker prediction, viral vector and biomanufacturing optimization, and personalized medicine.
How is AI being used with CRISPR?
AI is being used with CRISPR-based gene therapies to enhance the efficiency and accuracy of gene editing. AI models are being used to predict off-target effects and optimize target selection and delivery for more precise and effective genome modifications.
What Are The Current Challenges with AI?
The current challenges with AI in gene therapy include limited and heterogeneous data, the need for extensive computational power, data privacy and access concerns, labeling and annotation complexities, interpretability of AI models, generalization across datasets, and effective integration and fusion of diverse data modalities.
AI Disclosure: Feature image was generated by an AI image tool MidJourney.
Interested in how our AI models accurately optimize gene therapies, and optimize AAV vector design outcomes?
Download our biological validation reportReferences
- FDA Approved Cellular and Gene Products. FDA website. Content current as of 6/30/23. Accessed July 1, 2023.
- FDA approves novel gene therapy to treat patients with a rare form of inherited vision loss. FDA website. Content current as of 3/16/2018. Accessed July 1, 2023.
- FDA approves innovative gene therapy to treat pediatric patients with spinal muscularatrophy, a rare disease and leading genetic cause of infant mortality. FDA website. Content current as of 5/24/2019. Accessed July 1, 2023.
- FDA Approves First Gene Therapy to Treat Adults with Hemophilia B. FDA website. Content current as of 11/22/2022. Accessed July 1, 2023.
- FDA D.I.S.C.O. Burst Edition: FDA approval of Adstiladrin (nadofaragene firadenovec-vncg) for patients with high-risk Bacillus Calmette-Guérin unresponsive non-muscle invasive bladder cancer with carcinoma in situ with or without papillary tumors. FDA website. Content current as of 1/20/23. Accessed July 1, 2023.
- FDA Approves First Gene Therapy for Treatment of Certain Patients with Duchenne Muscular Dystrophy. Content current as of 6/23/2023. Accessed July 1, 2023.
- FDA Approves First Gene Therapy for Adults with Severe Hemophilia A. Content current as of 6/30/2023. Accessed July 1, 2023.
- Cell and Gene Therapy Fueled Large Surge in Clinical Holds Last Year. Published March 7, 2022. Accessed July 1, 2023.
- Shortening Gene Therapy Development Time with Strategic Use of Large Language Models. Form Bio Resource Center. Published March 2, 2023. Accessed July 1, 2023.
- Exploring the Versatality of Large Transformer Architectures and Their Implications for Gene Therapy Developers. Form Bio Resource Center. Published March 22, 2023. Accessed July 1, 2023.
- Karel Capek. R.U.R. A Fantastic Melodrama in Three Acts and an Epilogue. Published Jan 1, 1923.
- AI and Genomics: A Constructive 21st Century Affair. Colossal Laboratories and Biosciences website. Accessed July 1, 2023.
- Council of Europe website. History of Artificial Intelligence. Accessed July 1, 2023.
- The White House Office 2023 Meetings. May 18-19, 2023. Day 2: Artificial Intelligence (AI) Enabling Science and AI Impacts on Society. Access July 1, 2023.
- FACT SHEET: President Biden Issues Executive Order on Safe, Secure, and Trustworthy Artificial Intelligence. White House website. Published October 30, 2023. Accessed November 15, 2023.
- Gene Therapy Report Q4 2023- Q4 2027. CVS Health website. Published November 13, 2023. Accessed November 15, 2023.
- What the FDA’s New Safety Draft Guidance for AAV Gene Therapies Means and How to Be Ready. Published March 15, 2023. Accessed July 3, 2023.
- Cell and Gene Therapy Manufacturing Costs Limiting Access. Published February 21, 2023. Accessed July 1, 2023.
- Maurya, S., Sarangi, P. & Jayandharan, G.R. Safety of Adeno-associated virus-based vector-mediated gene therapy—impact of vector dose. Cancer Gene Ther 29, 1305–1306 (2022).
- Fourth Boy Dies in Trial of Astellas Gene Therapy Candidate. Genetic Engineering and Biotechnology News. Accessed July 1, 2023.
- 'Pull every lever': Marks doubles down on urgency to improve gene therapy manufacturing. Endpoints News website. Published November 6, 2023. Accessed November 16, 2023.
- Solving Gene Therapy Product Development Challenges Through Analytical Standardization. Form Bio Resource Center. Published November 8, 2023. Accessed November 16, 2023.
- IBM website. Federated Systems. Last updated April 13, 2021.
- Developing Machine Learning Powered Solutions for Cell and Gene Therapy Candidate Validation. Form Bio Resource Center. Published December 2022. Accessed July 5, 2023.
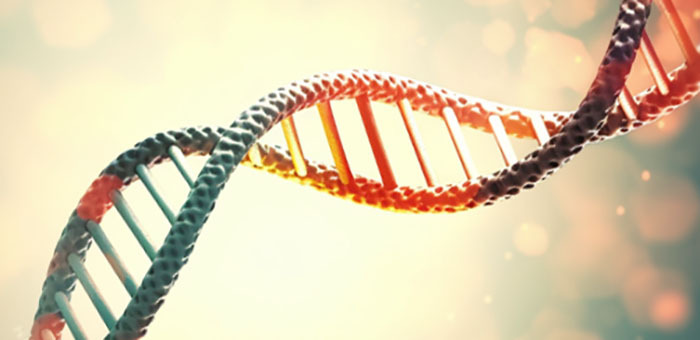